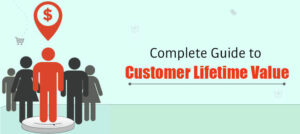
I have consulted hundreds of companies to plan their digital growth. While doing so, I observed that still a lot of startups/companies plan their projections wrong. Since they go with a basic calculation of Cost of Acquisition (or say a cost of conversion) and try to extrapolate it with unit sales targets (or say revenue). But while doing so, they either ignore or miss to calculate or determine the customer lifetime value. Which probably can have a direct impact on strategy and budgets.
What is Customer Lifetime Value (CLV)?
In simplest terms, customer lifetime value is the expected value creation by the same customer in due course of time. It basically is determined by the repeat buying or renewing of license etc by customers in due course (or say life expectancy of that customer for the respective product segment).
Why does Customer Life Time Value Matter?
Customer Time Value analysis is very much important to any business type. Be it an eCommerce company, An App (for in-app purchases), a B2B business (contract renewal, maintenance & services, etc). With the business becoming more competitive and buyers getting more intelligent; the acquisition costs are going up & above.
For businesses to sustain & to remain profitable they need to ensure that their Customer Life Time Value is higher.
Let’s Learn Customer Life Time Value by Examples:
So, let’s take an example here:
Let’s say if we take an example of an Antivirus company called QuickHeal (No endorsements here.. it just came on top of recall, thanks to their push on advertising :D).
So, let’s say if their antivirus license cost is Rs 1000 per year per installation. And let’s assume that due to higher CPC’s the cost of acquisition for them is Rs 1200 for every new customer.
It doesn’t sound profitable deal right? In fact, it sounds like a negative ROI.
Now let’s assume that the second year they manage to retain 50% customer for license renewal (i.e 5000 customers), and in a subsequent 3rd year again 50% of renewed customers continues (2500 customers). Finally, in the year 4th, no one further continues.
Year 1: Acquisition Cost = 1200 per customer
Total spending = Rs 12000000 (i.e. 10000*1200).
This is how a brand will calculate it:
Business in first year = 10000*1000 = 10000000
Business in second year = 5000*1000 = 5000000
Business in Third Year = 2500*1000 = 2500000
If we take an average of the average customer value here, it will be as below:
Total Revenue in 3 Years =17500000
Total Spending was = 1,20,00,000
Hence total business should be (revenue – expenditure) i.e. 17500000 – 12000000 = 5500000
Annual Contracts (as assumed in step 1) = 10000
Hence Average Customer Life Time Value would be: 5500000/10000 = 550
Which means probably quick heal can take into account 550 Rs as lifetime value of a typical antivirus license they sell. Now, accordingly, they can plan their further marketing budgets.
Although the first year was sounding negative, the lifetime value is still positive.
*Note: Above example is a raw example for explaining the concept. I haven’t taken into account any costs towards retention.
Now, if Quickheal decides to improve their Customer lifetime value, they would need to focus on either to ensure that customers stick to more time with them, or they should sell some add-ons to existing customers like say malware protection plan.
Relationship of Customer Life Time Value & ROI:
With the examples given above, it’s evident that:
[message type=”simple” bg_color=”#EEEEEE” color=”#333333″]Customer Life Time Value = Expected Life Time * Frequency of Repeat Buying * Unit Price[/message]
Expected Life Time:
Based on the product line, a lifetime of a customer can be defined. For example: For an eCommerce player like Amazon, the lifetime value of a customer can be very much prolonged. As there is a huge product line which can be catered to the users at varying stages of life.
On the contrary, if we talk about another eCommerce example of say ‘BabyOye’ or ‘FirstCry’ probably their Customers Life Time can be little lesser as compared to Amazon. As the products cater to kids up to defined age group. Afterward, users may not buy it. Well, it holds true, unless the brands have certain other defined KPI’s with respect to Net Promoter Scores (NPS).
How to Increase Frequency of Repeat Buying (Cross Sell & Up Sell):
As discussed above higher the repeat frequency higher will be the LTV and more will be the ROI. But how to increase the frequency of Repeat Buying?
Repeat buying can be of a varied nature; as below:
- Seasonal
- Impulse-Based
- Up-Sell / Cross Sell
Although the above types can go hand-in-hand or are inter-dependent too. Let’s understand it by examples:
Seasonal or Time Based:
Say I have bought a T-Shirt from Jabong.com; and as winters are approaching, I might visit them again to buy a Sweater. This will be triggered by my previous good experience with them.
Other examples can be planning it based on product life. So, let’s say Mr. X has bought a Laptop which comes with 3 years warranty. Probably an email triggered promotion exactly after 3 days, to remind him for a new laptop with added features and exchange offer; might boost the sale.
Brands use email Campaigns or targeted advertising campaigns (custom audience) to the buyers.
Impulse-Based:
There are many eCommerce products which are sold based on impulse. For example – Laptop Stickers. The person may not have a need at all; although looking at those cute/crazy stickers that too for a low price tag is just unavoidable. And we tend to buy them. Again it’s very important o understand what product will create that right impulse to your customers.
So, based on historical buying, the right product placements/promotions respective customer should be done.
Upsell/Cross Sell:
Based on the buying tendency Or previous purchase history, a lot many upsell or cross sales are done. For example, One who has bought a smartphone might also go for the mobile cover. Someone who is buying a DSLR might also consider buying a lens in the future.
Or for an agency like us; a client who is focused only on Social Media can also be pushed for say SEO.
This can be well planned with triggered email newsletters and/or dynamic remarketing/retargeting campaigns.
How to Calculate your Customer Life Time Value?
The traditional method of customer lifetime value calculation is based on Historic data. It can be put as below:
[message type=”simple” bg_color=”#EEEEEE” color=”#333333″]Avg Customer Life Time Value = (Sale 1 + Sale 2+…… + Sale n) * Average Gross Margins.[/message]
However, the calculation is NOT as easy as it sounds. Because there are a hell lot of variables which play a role here. Especially for companies which have huge product lines, like say Amazon. For every product line, there will be a different behavior, life expectancy and by frequency.
So the question comes, is it possible to predict the behavior? and to extend, can we really predict the Life Time Value of a customer based on behavior?
Predictive Modelling of Customer Life Time Value:
Now let’s get a little deeper to understand how the Customer Life Time Value Predictions are done. So based on previous Historical data modeling, we talked about the traditional approach. But it’s difficult to take into account multiple variables like product lines, pricing, behavioral difference, sales cycles, decision-influencing factors, etc.
Hence it’s very important that the model should be tailored precisely based on your business segment. I liked the way Jean-Rene Gauthier has segmented the businesses into 4 quadrants in his blog.
Non-contractual | Contractual | |
---|---|---|
Continuous Purchase |
Example: eCommerce Buying Like Amazon or Jabong |
Example: Credit Cards |
Discrete Purchases |
Example: Event Attendence |
Example: Insurance Plans, Cell Phones |
Based on the which quadrant your business lies, the variables are defined and a model is created. The hypothesis is then being tested to see if those are considered driving the predictions right.
Use of Machine Learning in Predictive Modelling:
With the huge amount of data and larger sets of data variables; it becomes very complicated to analyze and predict the Customer Life Time Value. Hence Machine learning is being deployed nowadays to predict the CLV. Machine Learning can take into multiple variables of any segment like eCommerce; where variables could be Customer demographics (age, gender, location), purchase history, web/session behavior, returns history, paying appetite, bundled products, payment methods and so on.
Machine learning algorithm then Churn the data or do the regression to identify standard deviations and then do the predictions. So based on predictions future marketing plans can also be done.
Attribution Modelling Vs Predictive Customer Life Time Value:
Attribution modeling helps us understand the importance of various touch points in the customer journey. Although if the attribution model is churned & Optimized it can help improve the Customer Life Time Value.
You remember in the above discussion we derived a formula for Customer Life Time Value
CLV = (Sale 1 + Sale 2+…… + Sale n) * Average Gross Margins
Here the Average Gross Margins considers the total spends to gain the respective revenue. Now, these spends can be optimized if the right attribution model is in place.
Why Attribution Modelling helps in increasing Customer Life Time Value?
The attribution models tell us the weight of specific touch points in the customer journey (For example Last Click, First Click, Weighted Average, etc). Accordingly, marketers like us can plan:
- Prioritize the digital channels while budget allocation. For example – Investing on Google Adwords (as first click interaction) vs investing on emailers/remarketing/retargeting (touchpoints during journey etc).
- Attribution model can also help in segmenting different user segments and planning different models around each segment. So that we can get the best from each segment.
- Prioritize or focus the sales/services efforts on product/customer segments which are highly like to convert.
In short, attribution models can channelize your spends in the right direction and improve the conversion costs and hence the gross margins.
I believe there will be a lot many other aspects which you would also have experienced while working upon your Customers Life Time Value. Please contribute your thoughts and I will try to accommodate them in the article with due credits.
0 Comments